Key points summary
03:40
Dr Hardman discusses the shift in the education industry from a place of panic to more strategic and practical conversations about the effect AI will have on people’s roles in the short, medium and long term.
06:54
She goes on to explain how some industries can utilise AI for laborious and repetitive tasks that humans aren’t optimised to do, especially those areas concerning data analysis.
09:20
Hardman discusses the uses of AI in recognising patterns within data. She goes on to explain how this will allow L&D teams to analyse any correlations between the training initiative and the performance following it.
14:13
She explains how a training request to a delivered experience, pre-AI, could take nine months, but with the use of AI, it can be completed within nine minutes, highlighting the time this frees up to focus on more strategic jobs.
23:43
She advises understanding what the AI you’re using has been trained on, so you can fully and objectively analyse the output.
25:14
Dr Hardman underlines the need for humans in collaboration with AI, stating that it’s humans that train the machine, work out how to define the response and then decide on the quality of the output.
Conversation transcript
Robin Hoyle: Phil, I met you when you did a really rather marvellous keynote speech at the World of Learning Conference in October at the Nestlé Exhibition Centre in Birmingham. Welcome to the Workplace Learning Matters podcast. Just give me a little bit of background about how you ended up where you ended up.
Dr. Philippa Hardman: Yeah, thank you. Thanks for the feedback on the talk, it was a great event.
I think I'm quite unusual in that I've worked a lot in corporate L&D as a learning designer, learning scientist – whatever we want to call it – but I have an academic background, so I'm still an affiliated scholar at Cambridge University, where I did a post-doc many years ago.
I've been interested and been researching for many years about how humans learn and how we can use technology to help them to learn better. I think what started as a very academic research project, led to me being very interested in technology and how can we use technology to increase access to great learning. It just became more and more practical. I actually decided to move out of higher education and continue my research, but in the world of EdTech.
I transitioned into a Chief Learning Officer type role, where I helped to build EdTech products with a more evidence-based approach. Rather than just trying to sell products, we were trying to sell pedagogy, to sell student outcomes.
Then more recently, this question around if and how technology can help people to learn has evolved into a question that's very much focused on AI, because it's everywhere now. It's a continuation of 20-odd years of research, but I'm now focused more on this question of if and how AI might be able to help us, particularly and specifically in the area of workplace L&D.
Robin Hoyle: AI is obviously a pretty hot topic at the moment. We've had Rishi Sunak doing an extended job interview – sorry, an interview with Elon Musk posted on his X platform – and obviously the summit there. There seems to be three camps around AI at the moment.
There are the people who are really excited, but I'm not quite sure what they're excited about yet. Then you've got the people who are imagining that none of this is ever going to happen. Elon Musk talked about, “We're all going to live in a world without work” the other day. I was thinking, “I remember the paperless office”, which never quite happened either. There are things around that where people are being, I think, with some degree of experience, sceptical about new technology.
Then, of course, there's the third group, which is the people are thinking, “This is the end of the world as we know it”. Why do you think there's such a wide range of views about artificial intelligence? Because let's be honest, it's been around for a long time. We've just seen a new generation of stuff come through. Why that disparate view?
Dr. Philippa Hardman: I think as someone who is from the humanities, a historian by training, I think we see this. I think it's a very human reaction to something that raises existential questions and people tend to get quite extreme positions or quite varied positions. I think the reactions are a result of the fact that we just don't really have much—despite the fact that, like you say, it's been around now for 60 odd years, as long as DNA and space travel—the average human on the street doesn't know that much about AI and machine learning. There's a lot of space.
There's a vacuum of understanding, which means that people can make up their own versions of the future, which are varied. I think what there's less of is some concrete examples of what it means in the immediate term. We've got lots of people talking about, “Is it the future where there is no work, where robots do the work and we all live a life of leisure on a universal income”, or is it the beginning of the end of the world?
I think there's some interesting commentary going on about how actually, as we all become—and this is a generalisation—less religious, we are looking with these big existential questions, and it seems like AI is the medium through which we're exploring some of those. But I think generally, what I have seen, particularly from this education angle, is a shift from what felt like some initial panic and a movement to deny it's happening to more strategic conversations and practical conversations about what this means for your role in the short, medium, and long term. What impact could this have?
We've had studies come out now by organisations like the Boston Consulting Group who tell us that it's going to increase our efficiency by at least 40%. We're getting more and more data now, more awareness of what this actually could look like and what it might mean. I would expect the conversation to become less hyperbolic and more focused on some of the realities and the nuts and bolts of this situation as we move forward.
Basically, it just takes a year for us to settle down.
Robin Hoyle: And to get a bit more of a pragmatic view of what it might be able to do.
There's a report out that talks about 60% of sales tasks being done by generative AI within the next five years. That's just out by Gartner. I think there is clearly an understanding of, “This is going to impact work”. But where are those impacts most likely to be felt? These things are outside of the learnings, which we'll come on to in a minute. But from just the general world of work, what can we now do that we couldn't do pre-chat GPT or the variations thereof? What is it that we're able to do now, which is moving the goalpost a little bit about how we think about the tasks that people are doing day to day?
Dr. Philippa Hardman: I think we've got quite a lot of precedent here. If we look at the medical world, which obviously is full of people at work; what we've seen there, and I think this is a pattern that we would expect to see replicated elsewhere, we've seen the automation of tasks. One is stuff that is very structured and very repeated. You maybe automatically think about a production line, but it might also be a process, for example, sales or taking customer questions, things that tend to have repeated patterns are those which will be automated first.
I work with organisations to identify what can we do tomorrow; what parts of our process can we automate and what shouldn't we? I think those things are always the things which are repeated or very laborious and data-based. In the medical industry, we've seen a lot of automation of very basic administrative tasks, but there's also things like reviewing scans, which require very close attention to detail, with a lot of time and a lot of energy.
Those tasks can be done more effectively by AI because it doesn't get tired. It hasn't got other things to do, and it’s trained to do one thing and one thing only. I think the jobs most likely to be automated are those which are very mechanical, very basic or very repeated, but also those which humans are not optimised to do, particularly those concerning data.
Robin Hoyle: One of the things that you said at the World of Learning conference was that AI was really good at spotting patterns. I guess that obviously speaks to the reading scans. There was that whole thing around using AI with radiographers, with mammograms, for example, looking at how efficient it was at being able to spot those anomalies that maybe a human being could miss because of a lapse of concentration or something not quite fitting what they were expecting.
There's that idea of the AI being really good at identifying the patterns. When it comes to the world of learning, where are the patterns where AI is uniquely positioned to help people to identify what they could do differently or what needs to happen from a learning perspective?
Dr. Philippa Hardman: This is what I've been looking into in the past couple of months or so. What does this mean in the context of L&D? I think one thing that we do have in L&D is a lot of data. One area that I've been exploring, which looks to have a lot of potential, is in the needs analysis space. Being able to spot patterns in learning interventions which did or didn't lead to something as simple as attendance. But we might also be able to start to triangulate data.
For example, to see, “Ah, hang on, these particular employees did this particular training, and we saw their performance increased by X amount over time”. We can input data into the machine about the training that we've already designed and the performance of our employees and see if there are any correlations there. That gives us new insights that we just haven't had before about what to design and when and how and for who. I think there are other areas where the ability of AI, not necessarily just to look for patterns, but also to summarise information, can be incredibly powerful for L&D.
One of the areas where we've all struggled historically is keeping up to date with how on Earth to design and deliver training. We default to this sage on the stage, off the shelf, or we build our own thing, or we have an event. But fundamentally, all of those things tend to focus around an expert talking, and then there being some opportunity to ask questions or a test to recall something. I think one thing that's really exciting and powerful for L&D professionals is the fact that they can access research for free to help them make decisions about the best course of action. For example, training online or in the flesh? What's the best approach to teach this thing to this human being? What's the best approach and the best pedagogy? I think that's been locked behind ivory towers for a long time and paywalls. That's incredibly powerful.
Then there's much more practical things. It can just make us much more efficient at creating stuff that we were creating before, but faster, which frees up time to do more strategic and more important jobs.
There's stuff that we can automate for efficiency and stuff that we can do, like the research, that augments, rather than automates what we do.
Robin Hoyle: That’s really interesting. I was much moved by an 18th-century economist called Fernando, who worked at the Bank of England. He came up with a thing called Fernando's Law of Comparative Advantage. Now, he was talking about countries. They were saying, “This country over here ought to be doing this thing, because actually they're really good at doing that, whereas we could concentrate on doing this”. But actually, it works in organisations as well, doesn't it?
For example, I do know how to do fancy animations on PowerPoint, but so do lots of other people. What they can't do is write the stuff that's in the PowerPoint to start with. It's just speeding up some of those things which are occasionally quite nice to do on a Friday afternoon when your brain's shot and you can play around. That can be quite cathartic sometimes. But nonetheless, it's not maybe the best use of everybody's time. If you're a learning designer or a subject matter expert, you ought to be using that to think more strategically, as you say.
I think one of the things that you mentioned was that idea of being able to summarise what's happening and to be able to say “We have evidence”. One of the podcasts in this series was with Professor Robert Brinkerhoff, who came up with a Success Case Model, which is basically what he has been doing since the 1970s. It’s basically saying, “Okay, let's have a look at these programmes. The programme is exactly the same. Why did these people change what they did, and these people over here didn’t? What else was going on? Was it that the trainer was having a bad day or was it something about the environment that they went back to?”
So, how does that move into the workplace? We've got an understanding and we maybe used AI to help us to decide it doesn't need to be online, but an event, or this doesn't need to be an event, this needs to be something else. How does that move beyond that intervention to say, “Here's some stuff”, and into, “Let's have a look at how you're using the stuff that we gave you earlier.”
How does AI bridge that gap a little bit? Because I think within the L&D industry, we're really quite bad at doing that.
Dr. Philippa Hardman: I've been looking at this through the angle of using the most established model for designing, like the Addy model. Yes, it can definitely help us at this analysis stage, where we're essentially generating and analysing and theming data. Great. But like you say, what next? What if we do realise that much of what we do as L&D professionals isn't as effective as it could be. We do know that for a fact. I think I shared that the World of Learning—it’s a quite depressing fact—but the average rate of transfer to on-the-ground application in the workplace from a learning experience is, at best, 12%. Most of the stuff that we do doesn't translate.
How do you bridge that gap from knowing what you've got to design, to designing it, to actually delivering it? I think there's all sorts that AI can do at the design and the delivery stage as well. As I say, there is a lot that we can do when using AI to help us to identify the best way to design something. Then there's also lots of tools out there which enable us to create the content much more rapidly.
What this means, of course, and this is something that I'm working with at the moment with a number of L&D teams to see how far we can push this, but if those processes are much faster, what it means is that we can do more of it. This might be disappointing. It might be that like, no, let's just put our feet up, but there is a scenario in which now, where it used to take me nine months to go from a training request to a delivered experience, it now takes me nine minutes.
What that means, partly, is that I can still have a cup of tea, but I can also then create 30 different learning paths, differentiated by department, differentiated by team, maybe even at the individual level. That's where things, I think, get very interesting with AI. It's almost like we've talked a lot in the past about personalised learning. Part of the problem for me is that a lot of the real promise of AI has already been promised through a lot of hyperbole about the potential of technology. We've talked a lot about personalised learning, so I'm now having to call it hyper-personalised just to differentiate it.
But what I mean is, everything has been disrupted already by AI. That might be a slight exaggeration, but if you think about Netflix, TikTok, music, food, TV, whatever, it knows what you like. It gives it to you at the point of time where you are most likely to want it. It nudges your behaviours. It does these things in the flow of your life. I think that's where AI gets interesting. This concept of being able to analyse needs, design something that is hyper-tailored and then deliver it in a way that is dynamic.
If I listen to Spotify and I say, “No, I don't like this thing”, it automatically changes it to better meet my preferences. It's got to the point now where it's better at predicting what music I like and want to listen to than I can. I think that's potentially the direction that this will eventually go in. But again, it's an interesting point.
One point we talked about at the event in Birmingham is that we've had these technologies around for 60-odd years. We've had these technologies that can be commercialised in a way that Netflix, Spotify, TikTok, whatever, commercialise them, for the best part of a decade, but it's not changed anything. I think the question is less around, can the technology do this? Can we build technologies to enable us to do this? We absolutely could. The question is, do we want it or are we empowered to? Or are we tied into processes and cultures, but also products like LMSs that just won't allow us to? Processes that don't give us the freedom and the space that we need to be able to do this.
I think that is a question for the industry and for people who build products. Are we just going to keep building the thing that sells? At the moment, if I wanted to build an AI product that sells, I would build one very similar to those which we've seen built over the last eight or nine months; tools that speed up what we already do. Because people buy when they're in pain. They don't necessarily buy things that are going to change everything, because that's longer term. We kick that into the long grass. I think it's a big question about culture and infrastructure and technology. But there's no doubt that tech could change everything. It's just…do we want it to?
Robin Hoyle: Going back to your Spotify analogy, and I think one of the things that works there is that it collects data. We talk about this idea of machine learning. It learns what you like, and sometimes that requires you to be active within the system. I'm constantly saying, “No, I hate that track”, or whatever it is. In my case, for some reason, every playlist it generates for me includes Iggy Pop, The Passenger, and Golden Brown by The Stranglers. I have no idea why. I still can't look at it.
The fact that they’re still there just means that they keep coming back again. It's gathering this data and saying, “Here's the stuff that you like”. But when we then link that into the world of work, within the flow of work, we don't always have that data. We don't have it in the same way.
You and I have spoken before about this idea of being able to give us pointers to what we've done and how what we did could have been different, and one would hope better, as a result of that. How does that work? What needs to change within the way we set up that transfer piece, which is pretty embarrassingly low. How do we set up the world of beyond learning to enable us to gather the data that you're giving to your Spotify and I'm giving to my streaming services?
Dr. Philippa Hardman: It does require a change on infrastructural levels and on a political level. There are big questions here about data. Who owns data? The thing with AI is, the more data it has on an individual level, the more powerful it becomes. There's an interesting ethical question here about how much of ourselves we want to give away in order to empower ourselves in the workplace. We do this already every single day, with our supermarket club cards and our Spotify and whatever else.
It's not a new question, but it's a new context for that question. There is a scenario in which we use those technologies to do things like track—we have stats on things like completion or quiz scores, but we all know this isn't really a measure of impact. So, what is? I think there is something about connecting together and tracking real-time data about the relationship between an action or an intervention and a behaviour.
On the ground, I'm working with quite a few sales teams. If we give them a certain learning intervention, how long does it take to impact on the rate of sales? Or does it? Does it have any impact whatsoever?
Then what are the variables that led to the impact? But that requires us to gather an awful lot of information, not just on our performance, but also on our behaviour. The technology is very capable of this. It's a question of whether we want it. I mean, you can imagine a scenario in which AI looks at my diary and sees I've got a big presentation tomorrow, and it asks me to stick in my slide from the last presentation, and it gives me feedback immediately in the flow of work on how to make it better for tomorrow.
But what that requires is for me to give it permission to look at a diary, to track how I write things, et cetera. I think, again, it's another example of, we could do it tomorrow with the investment and the right technology, but do we want it?
Robin Hoyle: I like the idea of the PowerPoint piece, but then there's a thing which is that the machine learning needs to learn what good looks like and what worries people. To a certain extent, I've seen some outputs that people have created from ChatGPT that are really poor because they've asked the wrong prompt question. We've got a mechanism there that says, “This is what good looks like”, but we don't want a cookie cutter presentation. We don't want something that looks like everybody else's presentation because everybody's using ChatGPT or its equivalent to generate this. How do we get that stuff in the machine, if you see what I mean?
Dr. Philippa Hardman: It's an interesting question. I often do this live demo where I share my screen and I go on ChatGPT and ask it to design me a course. Because AI is just trained on the internet, it just gives me back what is an average course design, which, of course, is content in a quiz — which is exactly what I don't want.
One thing I would say to anybody using AI is to make sure you understand what it's been trained on. Be aware that it is typically an average. We are seeing more and more AI tools emerge that are specialised. For example, there’s a tool called Elicit that's only trained on academic research papers. You can have an amount confidence in what it gives you. It's not just an average Joe's opinion, it's a specific specialism. I think we're seeing that more and more in the workplace. What we're seeing is lots of organisations building their own ChatGPT based on their own documentation and data, which can be helpful, but it’s also not a very powerful use of AI.
It's essentially another way of categorising and finding information through a chat interface, but it creates a space for stuff. We’re going to have to get better. One thing we need to get better at is we're going to have to build better language models that are not just generally trained on the internet. I think we'll see more specialised language models that are trained on expert data. But this just underlines the need for humans.
There’s this idea of the centaur or the cyborg that is part AI, part human. The most important part of that centaur is the human bit, because that's the bit that writes the prompt and validates the output. It's the humans that decide what to train the machine on, how to define it, and then decides on the quality of the output. I don't know if you saw, but this week, OpenAI have released AI Assistant, which enables anybody to build a chatbot, and everyone's getting very excited because now we can put in information and build a version of ourselves. But the same risks still apply.
It's still only as good as the prompt that you give it and the information that it's reading.
Robin Hoyle: Yeah, I had a conversation with somebody where I was saying, imagine for the moment that you've got a 16-year-old who's going to start college. You've decided that—because you've been a very responsible parent and you've kept them on the home computer so that you can track what they're doing—now is the time where you're going to get a laptop, because they're starting college. You go to your friendly IT person in your organisation and go, “Well, which laptop should I get them?”
A good IT person is going to turn around and say, “What do they want to do it for?” “Well, they play a few games, and they do this, and they watch some videos, but mostly I want them to have it for their coursework” and all these sorts of things. They will give you some advice. Now, instantly, you've got some bias there. Is your IT guy a Mac person or are they a PC person? Because never the twain shall meet. You get that bias in there right from the start.
Do they know how much budget you've got? And instantly, as a human being, you would have a conversation with that other individual and then decide which bits of advice they give you that you're going to follow or ignore. The phrase that's used frequently is this idea of these tools being your copilot, which is to say, knowing what you know about what they've given you and the prompt that you gave them and your own experience to this point, which bits are you going to take forward? Which are you going to edit? Which are you going to discard? That seems to me to be just a normal thing to do, but somehow people believe in the tech Gods. Therefore, it must be right, because ChatGPT told me it was.
How do we get trained people to work within this environment in which artificial intelligence is going to be part of their daily routine?
Dr. Philippa Hardman: I think you raised a really important point there that it's not any different from how we would deal with any information sources. We should always ask, “What's the quality of this? What do we think of it?” If we very quickly use Google, for example, in the flow of work, as we so often do, we don't just blindly take the first answer. I think this goes back to your first point around the dialogue around AI in quite an unhelpful way.
There's a lot of people who are just keen to point out when AI makes a mistake. It's just a machine that reads information and then gives it back. It can restructure it, which makes it different from the internet, but it's still just information and you are the expert. I think it's interesting.
In the education context, when I'm working with my students, we have a manifesto that very clearly says, AI is a tool for you to use in the same way that we'd use any other tool, but never trust it and always validate it. If you're going to use this to write your essay, then that's great. In fact, I require it, but I want to know what you got out of it and how you know it's reliable, how you prompted it, and what came out the other end. I think it's just an education piece around trying to get people into this mindset. AI is more like an apprentice than it is a professor. They need you to tell it what to do, how to do it, and then you need to check its work, because you know a lot more than it.
Robin Hoyle: Really interesting ideas. Phil, Dr. Philippa Hardman, thank you ever so much for your contribution to the Workplace Learning Matters podcast. I've really enjoyed our chat, and I think lots of other people will as well. Thanks ever so much for joining us.
Dr. Philippa Hardman: Thanks for having me.
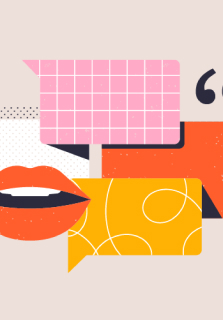
Get the thing here
Discover how a software company boosted sales by 50% through SPIN sales training, along with more success stories, in our free download: SPIN Evidence of Return on Investment.
Tell us your perspective
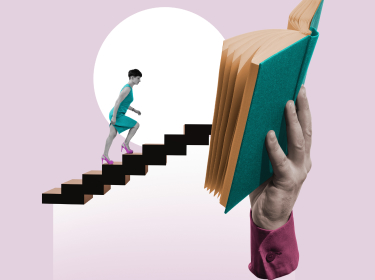
Sign-up and never miss an episode, perfect for busy people who don't have time to listen
Featured Video
Sign up to our newsletter
Sales, negotiation and communication insights direct to your inbox.
All the latest blogs, research and podcasts from Huthwaite International.